Essential Insights into Analytics and Business Intelligence
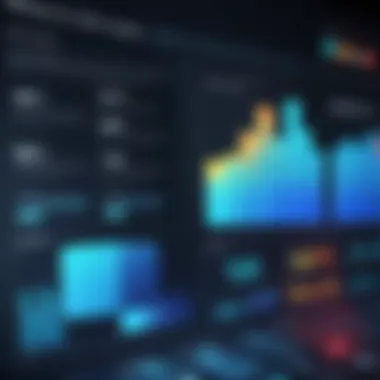
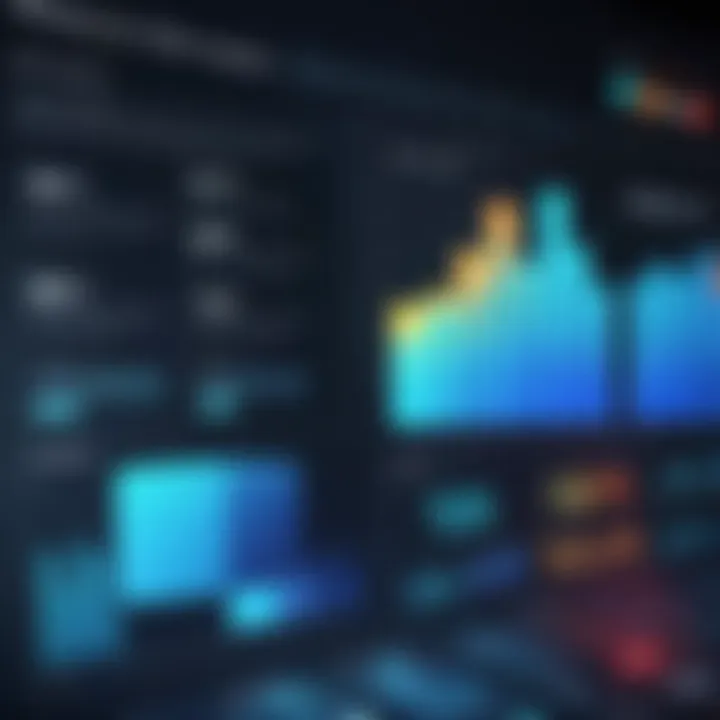
Intro
In an age where data is ubiquitous, the ability to analyze and derive insights from it has become essential for businesses. Analytics and business intelligence platforms play a pivotal role in transforming raw data into actionable information. These tools not only help organizations make informed decisions but also streamline operations and foster growth. Understanding how these platforms function, their core features, and their impact on decision-making is crucial for IT professionals and decision-makers alike.
The demand for data-driven decisions has surged, prompting organizations to invest in effective analytics solutions. Companies are now more aware that mere data collection is insufficient. They need sophisticated tools that can process data and reveal trends, opportunities, and potential pitfalls. This article aims to demystify the landscape of analytics and business intelligence platforms, detailing their functionalities, key features, and best practices for utilization.
We will also explore ethical considerations surrounding data use and highlight emerging trends in the industry. By synthesizing this information, the goal is to equip readers with a formidable understanding of how to leverage analytics technologies strategically.
Here we begin our exploration.
Prologue to Analytics and Business Intelligence
In the ever-evolving landscape of modern business, the ability to harness and interpret data effectively cannot be overstated. Analytics and business intelligence (BI) platforms serve as essential tools that empower organizations to make informed decisions based on robust data analysis. These systems offer insights that are critical for maintaining a competitive edge, optimizing operations, and enhancing customer engagement.
Understanding analytics and its relation to business intelligence is crucial. While both concepts aim to transform raw data into meaningful insights, they do so through different lenses. Analytics often focuses on statistical rigor, enabling deeper understanding and prediction of trends. On the other hand, business intelligence provides the necessary context for decision-making by reporting on historical data and current performance indicators. Together, they create a comprehensive framework for businesses seeking to improve their strategic capabilities.
Defining Analytics
Analytics is often described as the systematic computational analysis of data or statistics. It involves the use of sophisticated tools and techniques to analyze data sets for patterns and relationships that might not be immediately apparent. In practical terms, analytics can take many forms including descriptive analytics, which provides insights based on past events, and predictive analytics, which forecasts future occurrences based on historical data.
The importance of analytics in business cannot be minimized. It drives decisions that enhance performance and efficiency. Firms can leverage analytics to not only understand customer behavior but also to forecast market trends, thereby optimizing their strategies accordingly. Thus, defining analytics as more than just number crunching is vital; it is about enabling businesses to make data-driven decisions that lead to tangible outcomes.
Understanding Business Intelligence
Business intelligence is a broader term that encompasses various technologies, applications, and practices for the collection, integration, analysis, and presentation of business information. This process helps businesses make better-informed decisions. Essentially, BI systems gather past and current data, often from multiple sources, to provide a coherent view of organizational performance.
The role of BI is particularly pronounced in reporting and visualization. Organizations can generate dashboards and reports that encapsulate key metrics and KPIs, allowing stakeholders to see the bigger picture at a glance. Moreover, tools within BI platforms facilitate querying and exploration of the data, which aids in drawing actionable insights. By harnessing the power of business intelligence, organizations can respond strategically to market demands and improve operational efficiency.
Understanding the nuances of analytics and business intelligence is not just beneficial; it's essential for business survival in a data-driven world.
The Role of Data in Analytics and Business Intelligence
Data serves as the foundation of analytics and business intelligence. Understanding how to leverage data is essential for organizations aiming to make informed decisions. The insights derived from data analysis can drive strategies, enhance operational efficiency, and improve overall performance. Businesses today are inundated with various data types from different sources, and recognizing the significance of data in this realm is vital.
Types of Data
In the context of analytics, data can be categorized into several types.
- Structured Data: This type is highly organized and easily searchable. It usually resides in relational databases and can be mapped into predefined fields. Examples include numerical values and dates.
- Unstructured Data: Unlike structured data, unstructured data lacks a specific structure. Examples are text files, social media posts, and images. Analyzing this data often requires advanced tools and techniques.
- Semi-Structured Data: This data does not fit into a rigid structure but contains tags or markers to separate data elements. JSON and XML are common examples.
Each type has unique properties and advantages that can be utilized in analytics, making it crucial for organizations to identify the forms they possess.
Data Quality and Integrity
Data quality is a critical aspect of analytics and business intelligence. High-quality data ensures that analyses are accurate and reliable.
- Accuracy: Data should reflect the true values or facts it describes. Any inaccuracies can lead to misguided decisions.
- Completeness: This aspect examines whether all necessary data is present. Missing data can skew results and insights.
- Consistency: Data must remain uniform across various sources. Inconsistencies can arise when data is gathered from different systems, leading to potential errors.
Ensuring data integrity involves maintaining the authenticity and reliability of data, which is essential for any analytical process. Organizations must implement robust measures to cleanse, validate, and maintain their data. If data integrity is compromised, the results derived from analytics may be fundamentally flawed.
"The importance of data quality cannot be overstated; it is essential for achieving optimal performance in analytics and business intelligence."
In summary, understanding the role of data in analytics and business intelligence is fundamental to harnessing its full potential. Organizations must prioritize data types and quality metrics to derive meaningful insights that align with their strategic goals.
Key Features of Analytics Platforms
Analytics platforms serve as the backbone of informed decision-making in organizations. Their features are essential in enabling businesses to analyze data comprehensively and derive actionable insights. In this section, we will discuss various key elements that are pivotal in understanding these platforms better, providing substantial benefits to users.
Data Visualization Tools
Data visualization tools are a core feature of analytics platforms. They allow users to create visual representations of data, making complex datasets easier to understand. Tools like Tableau and Microsoft Power BI provide interactive dashboards and charts that help organizations make sense of their data quickly.
The importance of data visualization cannot be overstated. It enhances communication between stakeholders, as visual data representations can convey messages more effectively than raw numbers or text. By utilizing these tools, businesses can identify trends, spot anomalies, and uncover patterns that may not be immediately obvious through traditional reporting methods.
Moreover, well-designed visuals can lead to quicker, more informed decisions. Companies leveraging visualization tools often experience improved collaboration among teams, as everyone can access the same data in a format that is intuitive and engaging. The result is a data-driven culture that fosters innovation and strategic planning.

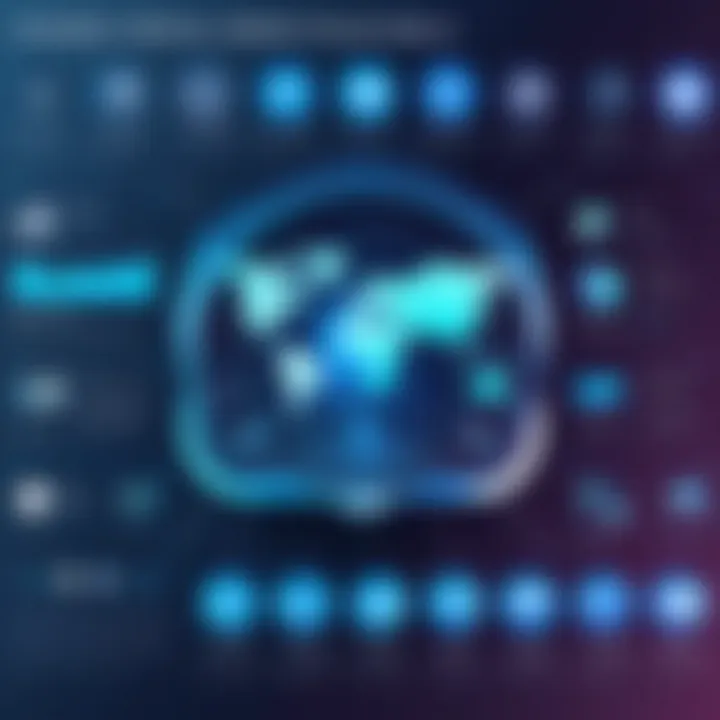
Predictive Analytics Capabilities
Predictive analytics capabilities are another crucial feature of modern analytics platforms. These capabilities employ statistical algorithms and machine learning techniques to analyze historical data and predict future outcomes. This proactive approach can significantly enhance business strategies and operational efficiency.
With predictive analytics, organizations can anticipate trends, optimize resource allocation, and proactively address potential challenges. For instance, retailers can forecast inventory needs based on past purchasing behaviors, thereby improving stock management and customer satisfaction. Similarly, financial institutions can assess risk levels more accurately, leading to better-informed lending decisions.
It's also worth noting that predictive analytics integrates seamlessly with other features of analytics platforms. When combined with data visualization tools, users can visualize potential future scenarios, making it even easier to understand complex predictions and their potential impacts on the business.
Real-time Data Processing
Real-time data processing is becoming increasingly important as businesses strive to remain competitive in a fast-paced market. This feature allows organizations to analyze data as it is generated, rather than waiting for batch processing. Platforms that support real-time data processing can provide immediate insights, enhancing responsiveness to changing conditions.
For example, businesses can monitor customer interactions in real-time, enabling instant decision-making. This agility can lead to improved customer service and engagement. Additionally, real-time data processing can enhance operational efficiency by allowing firms to quickly identify and respond to issues as they arise, rather than after the fact.
The ability to process and analyze data in real-time fosters a dynamic approach to business management. Organizations that invest in this capability can gain a significant competitive edge, positioning themselves as leaders in their respective industries.
"Real-time processing transforms the way businesses operate, enabling immediate adaptation to market shifts."
Comparative Analysis of Leading Platforms
In the evolving domain of analytics and business intelligence, conducting a thorough comparative analysis of leading platforms is essential. Businesses face a multitude of options, each offering distinct capabilities, interface designs, and integrations. Organizations must understand these differences in order to make informed decisions that align with their strategic goals. This section will delve into top business intelligence solutions, explore emerging players in the market, and provide case studies on platform implementation to highlight how these platforms perform in real-world scenarios.
Top Business Intelligence Solutions
Established platforms such as Tableau, Microsoft Power BI, and Qlik Sense dominate the field of business intelligence. These tools provide a mix of functionalities, catering to both analysts and decision-makers.
Tableau stands out for its intuitive interface and strong data visualization capabilities. It allows users to create interactive dashboards easily, which can uncover insights through visual narratives. Its ability to connect to various data sources enhances its flexibility.
Microsoft Power BI integrates seamlessly with Microsoft products, offering a user-friendly experience, especially for those familiar with Excel. Its robust analytical tools and sharing capabilities make it a popular choice amongst businesses looking for affordability without sacrificing quality.
Qlik Sense, on the other hand, provides a unique associative model, allowing users to explore data freely rather than being limited to pre-defined paths. This freedom fosters deeper insights, making it ideal for complex data analysis.
These platforms represent more than just tools; they serve as ecosystems where data-driven decision-making thrives. However, it is also important for organizations to evaluate how these tools fit their existing tech stack.
Emerging Players in the Market
While established brands lead the market, several emerging players are gaining traction. Tools like Looker, Sisense, and Domo offer innovative features that cater to niche markets or specific business needs.
Looker is recognized for its data modeling capabilities and its strong focus on collaborative analytics. By allowing teams to share insights effortlessly, it encourages a data-centric culture within organizations.
Sisense embraces a more technical audience, blending analytics with cloud capabilities. Its ability to handle large data sets and provide dense insights on-demand sets it apart.
Domo provides a comprehensive solution by combining BI capabilities with data visualization in a single platform, emphasizing real-time data and business applications.
These rising stars illustrate the diversification within the market, giving businesses more choices tailored to their particular analytics strategies.
Case Studies on Platform Implementation
Analyzing real-world case studies is crucial to understand the practical applications and outcomes of various platforms. For example, a retail chain implemented Tableau to visualize sales performance across locations. They found trends quickly and adjusted strategies to boost underperforming areas, resulting in a 15% increase in overall sales within six months of deployment.
Similarly, a tech company adopted Microsoft Power BI to improve reporting efficiency. Their reporting process went from weeks to days, allowing for quicker decision-making. The integration with existing Microsoft tools fostered employee adoption, leading to a smooth transition.
To summarize, businesses must weigh the strengths and weaknesses of each platform relative to their needs. A tailored approach can result in meaningful improvements in analytics strategy and business performance.
Implementation Strategies for Businesses
Implementing analytics and business intelligence platforms is a critical endeavor for organizations aiming to harness data effectively. This section discusses the strategies necessary for successful implementation, exploring the various elements, benefits, and considerations need to improve performance and decision-making.
Assessing Business Needs
Before selecting a platform, it is essential to understand specific business needs. This involves clearly identifying the goals and challenges the organization faces. Stakeholders should collaborate to define how analytics can address these issues. Factors to consider may include:
- Current data sources and their quality.
- The types of reports and insights required.
- User access levels and data security concerns.
Through this process, organizations will gain clarity on requirements, allowing them to choose a platform that aligns with their objectives. This tailored approach increases the likelihood of adoption and ultimately enhances return on investment.
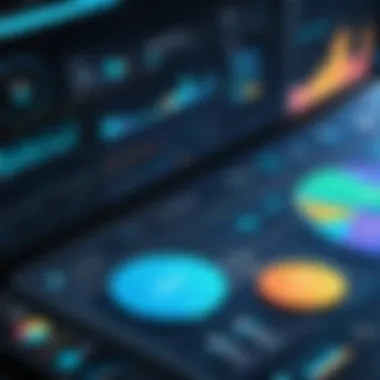
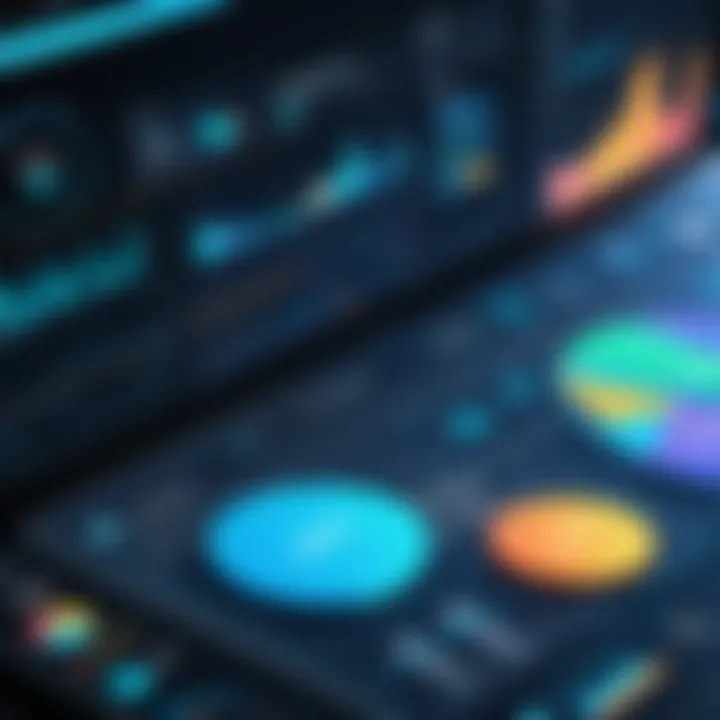
Platform Customization and Integration
Once a platform is chosen, customization and integration become vital steps in the implementation process. Off-the-shelf solutions often require adjustments to meet unique organizational needs. Customization may involve tailoring dashboards, metrics, and reporting structures. Moreover, integrating the new platform with existing systems is crucial. This integration allows for seamless data flow and consistency across various tools, such as CRM systems, ERP solutions, and databases.
Benefits of proper customization and integration include:
- Enhanced user experience, leading to higher user adoption rates.
- Improved data consistency, minimizing errors and discrepancies.
- Greater utility of analytics in everyday decision-making.
Training and Support for Users
Training users on the new platform is often overlooked, yet it is essential for effective utilization. A well-rounded training program should address various aspects:
- Technical training on using the platform.
- Understanding data interpretation and analytics.
- Continuous support through documentation and user forums.
Organizations should offer training sessions, either live or recorded, tailored to different user levels. Providing ongoing support is equally important in ensuring that users feel confident and capable of leveraging the platform for their needs. This commitment to user education will bolster the overall effectiveness of analytics initiatives over time.
"In the realm of business intelligence, the relevance of user training can never be overstated. Well-trained users can unlock the full potential of data-driven platforms."
Implementing these strategies effectively sets the stage for organizations to maximize their analytics and business intelligence efforts. By assessing needs, customizing platforms, and training users, organizations can navigate the complex landscape of business intelligence more successfully.
Evaluating Performance and ROI
Evaluating performance and return on investment (ROI) is critical for any business utilizing analytics and business intelligence platforms. This process allows organizations to measure the effectiveness of their implemented solutions and understand their contribution to overall business goals. The evaluation not only provides insights into whether the investments are yielding the expected outcomes but also helps to steer future investments and adjustments in strategy.
Metrics for Success
To gauge the success of analytics initiatives effectively, businesses must rely on specific metrics that align with their objectives. Commonly used metrics include:
- Cost Savings: Quantifying reductions in operational costs due to better decision-making and efficiencies gained through analytics.
- Increased Revenue: Measuring any growth in sales directly attributable to insights gained from analytics platforms.
- User Engagement: Evaluating how often and effectively internal users or customers utilize the analytics tools offered.
- Time to Decision: Assessing how analytics impact the speed of timely decision-making within the organization.
- Project Completion Rates: Determining if more projects produce results on time as a result of data-driven initiatives.
Understanding these metrics will allow organizations to benchmark their performance against their own historical data or industry standards. Regularly analyzing these figures helps in identifying areas for improvement.
Continuous Improvement Processes
Having metrics in place is just the first step. Organizations should focus on continuous improvement processes to ensure that analytics provide ongoing value. This involves:
- Regular Review Cycles: Establishing a routine for evaluating analytics performance and discussing findings among teams. These discussions should also include any challenges faced in maximizing the platform's utility.
- Stakeholder Involvement: Ensuring that all relevant stakeholders participate in these reviews. This helps gather diverse insights and fosters a culture of collaboration.
- Feedback Mechanisms: Implementing structured channels to gather feedback from users on the utility of the analytics tools. Understanding user experience can highlight areas needing enhancement.
- Training Sessions: Continuous education on new features or capabilities of analytics platforms can deepen user understanding and improve overall performance.
- Iterative Adjustments: Based on the reviews, stakeholders should make informed decisions to refine analytics strategies, tools, or approaches. This might involve adjusting algorithms, reevaluating data sources, or incorporating new technologies such as machine learning.
"The essence of evaluating performance lies not just in the metrics, but in the lessons learned from them."
This structured approach ensures that analytics and business intelligence remain aligned with organizational goals. By committing to evaluating performance and ROI, businesses can derive the full potential from their investments in analytics.
Challenges and Limitations
The realm of analytics and business intelligence platforms is not without its challenges and limitations. Addressing these factors is crucial for organizations seeking to maximize the benefits of data-driven decision-making. Understanding these challenges allows professionals to implement strategies that mitigate risks and enhance the effectiveness of analytics initiatives.
Data Privacy Concerns
In today's data-centric environment, data privacy has emerged as a pivotal concern for organizations that harness analytics. Various regulations, like the General Data Protection Regulation (GDPR) in Europe, impose strict guidelines on how businesses can collect, store, and process personal data. Failing to comply with these regulations can lead to substantial penalties and legal ramifications.
Moreover, as businesses gather vast amounts of consumer data, they must establish rigorous security protocols. Unauthorized access to sensitive information not only erodes consumer trust but also damages a brand's reputation. To navigate these complexities, organizations must invest in robust data governance frameworks that emphasize transparency, accountability, and ethical practices concerning data use.
"Organizations violate data privacy not just by neglect, but sometimes by misinformation about what data is safe to use."
Resistance to Change within Organizations
Implementing new analytics platforms often meets resistance from staff. This resistance can stem from various factors, including fear of job displacement, reluctance to adapt to new technologies, or skepticism regarding the effectiveness of analytics. Such barriers can slow down the adoption process and hinder the potential benefits that a new system could bring.
To combat resistance, it is essential for organizations to foster a culture that embraces change. This can be achieved through effective communication, highlighting the value of analytics in informing decision-making, and demonstrating how these tools support, rather than replace, human roles. Additionally, involving employees in the decision-making process regarding analytics tools ensures that their insights and experiences are valued, thereby enhancing buy-in and engagement.
Overcoming Data Silos
Data silos pose significant hurdles to successful analytics implementation. When different departments or teams within an organization hoard their data, it prevents a comprehensive view of the business landscape. These silos can lead to inconsistencies and misinformed decisions based on fragmented data sets.
To overcome these barriers, businesses must encourage a collaborative environment that promotes data sharing across teams. Establishing centralized data repositories can provide access to vital insights and foster interdepartmental communication. Furthermore, equipping teams with the right tools to visualize and analyze data holistically can help break down these silos.
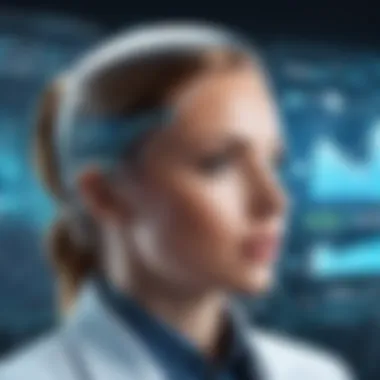
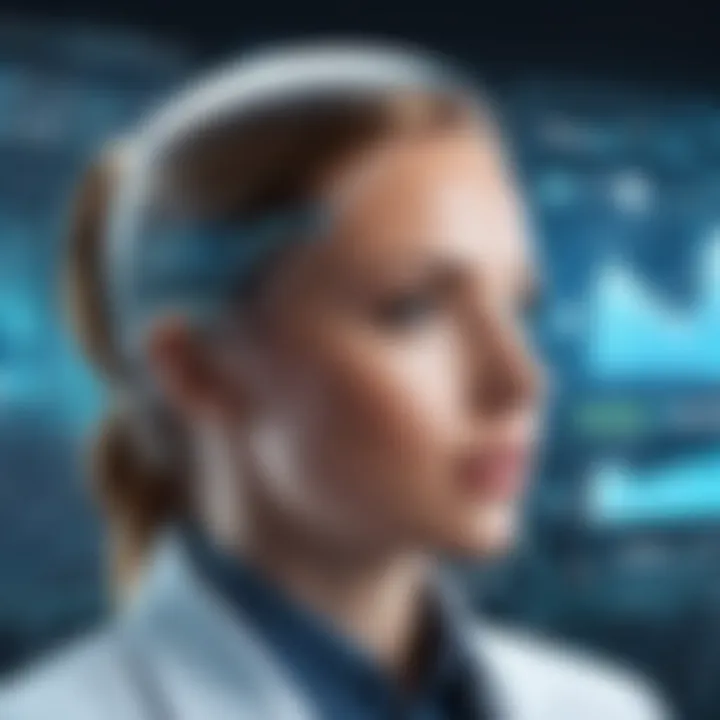
Ultimately, addressing challenges and limitations, including data privacy concerns, organizational resistance, and data silos, is essential for maximizing the potential of analytics and business intelligence platforms. By taking a proactive approach, organizations can overcome these obstacles and thrive in a data-driven world.
Ethical Considerations in Analytics
The importance of ethical considerations in analytics cannot be overstated. As businesses increasingly rely on data to make informed decisions, they must also take into account the implications of their data practices. Ethical usage of data protects not only the integrity of the organization but also the rights of individuals. Within this realm, two critical aspects come forth: the responsible use of data and the bias in data interpretation. These elements shape the landscape of analytics and are fundamental to fostering trust and accountability in data-driven environments.
Responsible Use of Data
Responsible use of data involves collecting, storing, and analyzing information in a manner that respects privacy rights and complies with legal standards. Organizations have an obligation to safeguard user data against unauthorized access and data breaches. This entails implementing robust security measures and adhering to regulations such as the General Data Protection Regulation (GDPR) or the California Consumer Privacy Act (CCPA).
- Transparent Data Practices: Companies should clearly communicate how data is collected and the purposes behind its usage. This builds trust with users and informs them of their rights regarding their own data.
- Informed Consent: Users should be given choices about what data they share. This empowers individuals and emphasizes the ethical commitment of the organization to respect user autonomy.
- Anonymization Techniques: Where possible, organizations should anonymize data to reduce the risk of re-identifying individuals when analyzing datasets. This mitigates potential harm and reinforces responsible data stewardship.
Adopting these practices leads to more ethical frameworks in analytics, fostering both innovation and trust in data-driven insights.
Bias in Data Interpretation
Bias in data interpretation is a significant concern in analytics, as it can skew findings and lead to misguided decision-making. Data itself is not inherently biased, but the ways in which it is collected, analyzed, and represented can introduce biases that affect outcomes.
Potential sources of bias include:
- Sampling Bias: When the dataset used in analysis is not representative of the entire population, conclusions drawn may be flawed and not applicable to broader contexts.
- Confirmation Bias: Analysts may intentionally or unintentionally seek out data that supports their preconceived notions, ignoring contradictory evidence. This distorts interpretations and can mislead stakeholders.
- Algorithmic Bias: Machine learning algorithms can perpetuate existing biases present in the data they are trained on, leading to outcomes that may be unfair or discriminatory.
"Without a critical lens on data interpretation, organizations risk making decisions that reinforce biases rather than eliminate them."
To address these biases, organizations should:
- Regularly audit and diversify data sources to ensure comprehensive datasets.
- Foster a culture of critical analysis among analytics teams, emphasizing the need for objectivity.
- Implement training programs to raise awareness of biases and how they can influence interpretation.
Future Trends in Analytics and Business Intelligence
Understanding the future trends in analytics and business intelligence is essential for organizations wishing to maintain a competitive edge. These trends not only reflect technological advancements but also the shifting landscape of business needs and customer expectations. As analytics continues to evolve, businesses must adapt their strategies to leverage the latest innovations effectively.
Key trends indicate an integration of more sophisticated technologies, which allow for better data management, analysis, and actionable insights. Organizations that ignore these shifts risk falling behind competitors who capitalize on advanced tools and techniques. It is crucial to comprehend these trends and their implications for successful decision-making processes in today’s data-driven environment.
Artificial Intelligence Integration
Artificial Intelligence, or AI, is becoming a cornerstone in analytics and business intelligence platforms. Its integration helps automate complex tasks, improves accuracy, and enhances decision-making capabilities. By utilizing AI, organizations can analyze vast amounts of data quickly, identify patterns, and gain insights that would be nearly impossible through manual processes.
Key benefits of AI integration include:
- Automated Data Analysis: AI can process and analyze data faster than traditional methods, allowing for real-time insights.
- Predictive Analytics: Algorithms can identify future trends based on historical data, guiding strategic initiatives.
- Enhanced Visualization: AI-driven tools can present data in more intuitive formats, making insights clear and actionable.
However, the use of AI also raises considerations regarding data accuracy and transparency. Organizations need to ensure that AI models are trained on high-quality datasets to avoid bias and inaccuracies in results. Moreover, understanding how AI arrives at certain conclusions is essential for maintaining stakeholder trust.
The Move towards Self-Service Analytics
The trend towards self-service analytics is transforming how organizations approach data analysis. This shift enables non-technical users to access and analyze data without needing extensive IT support. Platforms now offer user-friendly interfaces that empower business users to generate reports and dashboards on their own.
Benefits of self-service analytics include:
- Increased Agility: Teams can make data-driven decisions promptly without waiting for IT to create reports, leading to faster responses to market changes.
- Wider Adoption of Business Intelligence: Employees across different departments become more engaged and informed through easy access to analytics tools.
- Reduced IT Burden: IT departments can focus on more complex tasks rather than routine reporting.
Despite its advantages, self-service analytics requires a strong governance framework. Organizations need to ensure that users understand data interpretation and remain consistent in metrics and categorizations. Striking a balance between accessibility and data governance is critical to maximizing the benefits of this trend.
"The integration of AI in analytics is reshaping how organizations view data, transitioning from reactive decision-making to proactive strategies."
End
In closing, the exploration of analytics and business intelligence platforms unveils their pivotal role in shaping an organization's strategic decisions. These platforms are not just tools; they are essential components in understanding market trends, customer behavior, and operational efficiency. With the constant evolution of technology, businesses must recognize the value of integrating advanced analytics into everyday practices.
Summary of Key Points
- Integration of Analytics: Businesses must integrate analytics to harness insights that guide decision-making processes. This integration can lead to improved forecasting and enhanced operational efficiencies.
- Impact of Data Quality: The quality of data underpinning these systems is crucial. Poor data can result in misleading insights, emphasizing the importance of data integrity and proper management.
- Future Trends: As technology advances, the shift towards artificial intelligence and self-service analytics is becoming evident. Organizations should prepare for these changes to remain competitive.
"Data is the new oil, but like oil, it must be refined to be useful."
The Path Forward for Organizations
Organizations must adopt a proactive approach towards analytics. They should foster a culture that values data-driven insights, invest in training for staff, and prioritize ethical considerations in data usage. Collaboration across departments can enhance the understanding and implementation of analytics. Moreover, as self-service analytics rises, companies should ensure that employees have access to robust tools that democratize data insights.
In summary, the journey does not end with the deployment of analytics platforms. Continuous evaluation and adaptation are critical to unlocking their full potential. For organizations keen on maintaining a competitive edge, the commitment to evolving analytics capabilities will be a necessary step in the path forward.