Big Data and Predictive Analytics for Decision Making
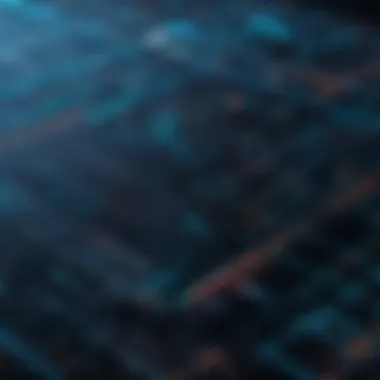
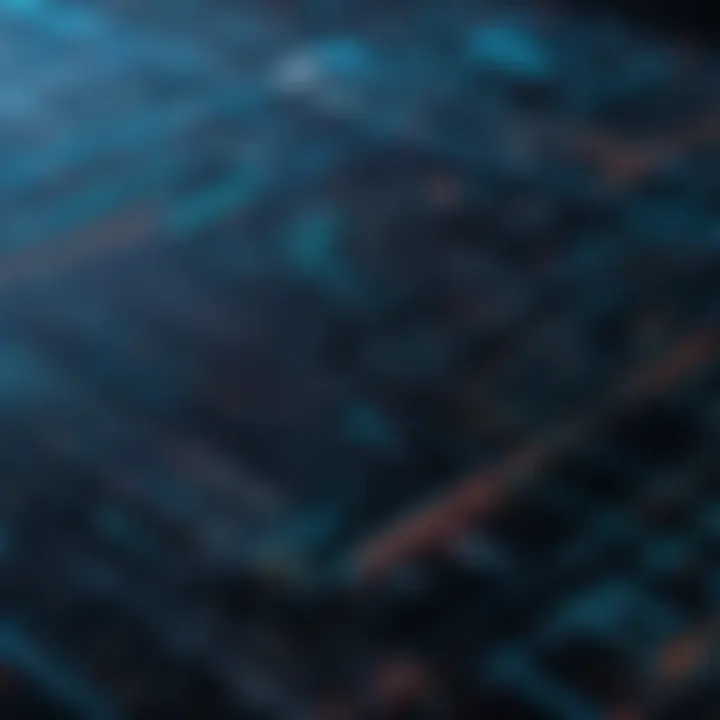
Intro
In the modern digital landscape, the terms big data and predictive analytics have become pivotal in shaping business strategies and decision-making processes. As organizations navigate an increasingly complicated environment filled with vast amounts of data, the ability to harness this information for predictive purposes becomes not just an advantage but a necessity. This narrative explores the synergy between big data and predictive analytics, unpacking their definitions, methodologies, and practical applications across various industries.
Big data refers to the substantial volume of structured and unstructured data generated continuously. This data can originate from numerous sources: customer interactions, social media activities, sensor data, and transactional records. On the other hand, predictive analytics utilizes statistical algorithms and machine learning techniques to analyze this data, enabling organizations to forecast future outcomes based on historical patterns. Together, they provide organizations with the tools essential for making informed decisions that align with market trends and consumer behaviors.
The significance of these concepts has escalated sharply in today’s technology-driven ecosystem. Industries such as finance, healthcare, and retail utilize these analytics to better understand their operational landscapes, reduce costs, and enhance customer satisfaction. With the volume of data expected to grow exponentially, professionals in IT and data science face an imperative to refine their skills in big data management and predictive modelling.
This article will explore the methodologies employed in predictive analytics, the challenges associated with managing extensive datasets, and ethical concerns surrounding data usage. Additionally, it will showcase real-world applications through various case studies, highlighting the effectiveness of predictive analytics in improving decision-making processes. As we proceed, the future trends that may influence this evolving field warrant careful consideration, as they have the potential to reshape industries at large.
Understanding Big Data
Understanding Big Data is crucial in the current digital age. It provides insights that empower organizations to make informed decisions, ultimately enhancing operational efficiency and competitive advantage. The ability to harness the massive scales of data being generated allows businesses to uncover trends, patterns, and correlations that were previously hidden. In this article, we will explore what constitutes big data, its defining characteristics, sources, and its implications in various fields.
Definition of Big Data
Big Data refers to the vast volumes of structured and unstructured data generated at high speeds from various sources. This data is too complex and large for traditional data-processing software to handle efficiently. It encompasses data from social media, sensors, transaction records, and more. The significance of Big Data lies in its potential; when analyzed properly, it can lead to valuable insights that drive business strategy and innovation.
Characteristics of Big Data
Volume
Volume is one of the primary characteristics of Big Data. It refers to the sheer amount of data being generated. With the proliferation of digital technologies, the data volume is growing exponentially. Companies collect data from different sources, leading to a notable challenge of storage and processing. Managing large datasets can be overwhelming, but the opportunities for analysis and insight make it a beneficial focus for this article. The large scale offers a unique feature: more data often translates into more accurate predictions and trends.
Velocity
Velocity relates to the speed at which data is generated and processed. In an increasingly connected world, data flows in at an unprecedented rate. This characteristic is important as businesses need real-time or near-real-time processing to remain competitive. Fast data processing can enhance customer experiences and operational responsiveness. The challenge, however, is maintaining the processing speed without compromising data quality.
Variety
Variety refers to the different types of data being generated. Unlike traditional data structures, big data includes text, images, videos, and more. This diversity of data forms allows for comprehensive analyses from various angles, providing a holistic view of the information at hand. Variety presents a unique challenge in terms of integration and analysis, but it also opens up new avenues for insights that are not available through standardized data alone.
Veracity
Veracity is concerned with the accuracy and truthfulness of the data. Given the vast amounts of data collected, ensuring its quality becomes increasingly critical. Poor data quality can lead to flawed analyses and misguided decisions. Therefore, maintaining high veracity is essential for reliable outcomes in any predictive analytics initiative. The unique feature of veracity is that it demands rigorous validation processes, which can be resource-intensive.
Value
Value signifies the importance and potential benefits obtained from analyzing big data. The data itself may not hold immediate value; instead, its value emerges when insightful conclusions are drawn. Extraction of value from big data allows organizations to enhance decision-making. The challenge lies in determining which data is worth analyzing and how to interpret it effectively.
Sources of Big Data
Big Data comes from numerous sources, each contributing significantly to the growing data landscape. Understanding where this data originates is key to leveraging it effectively.
Social Media
Social Media platforms such as Facebook and Twitter generate a substantial amount of data through user interactions and engagements. This data can reveal consumer sentiments, trends, and behaviors, making it a valuable resource for businesses. The downside is the significant noise present in social media data, which can complicate analyses.
IoT Devices
IoT Devices play a major role in producing big data. Devices connected to the Internet, like sensors and smart home devices, continuously collect data. This data can be utilized for predictive analytics in various applications, from smart cities to healthcare. However, the challenge is managing the vast amounts of data these devices produce.
Transactional Data
Transactional Data is generated from daily operations, including sales and customer interactions. This type of data is structured and typically easy to analyze, making it a fundamental component of big data strategies. The challenge here is maintaining data integrity and ensuring accuracy in the data collected.
Sensor Data
Sensor Data refers to information collected from various sensors. This data is often used in manufacturing, logistics, and environmental monitoring. While sensor data can provide critical insights, managing the continuous stream and ensuring its accuracy can present difficulties.
Understanding the multiple aspects of Big Data is essential for any IT professional or organization hoping to implement predictive analytics effectively. The characteristics and sources we’ve outlined set the stage for a deeper exploration of predictive analytics as we continue in this article.
Defining Predictive Analytics
Predictive analytics is increasingly important in today's data-driven world. It offers valuable insights that help organizations make informed decisions. Understanding how predictive analytics works is essential for harnessing its benefits effectively.
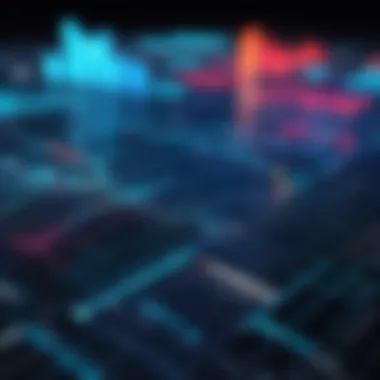
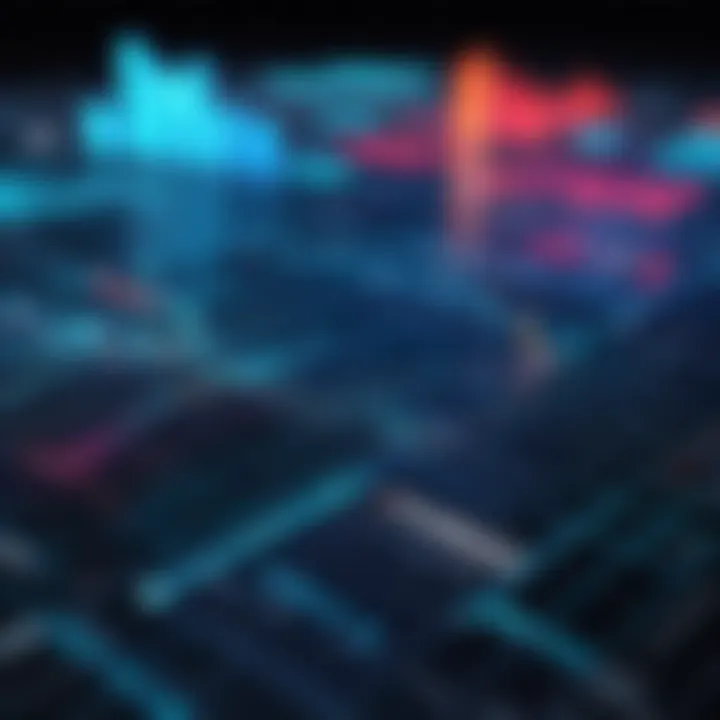
Overview of Predictive Analytics
Predictive analytics involves using historical data to predict future outcomes. This discipline blends statistics, machine learning, and data mining. It identifies patterns and trends, enabling analysts to draw meaningful conclusions. In various fields, predictive analytics is applied to forecast customer behavior, assess risks, and improve operational efficiency. Its relevance spans multiple sectors, making it a crucial topic in examining big data.
Techniques in Predictive Analytics
Statistical Modeling
Statistical modeling is a cornerstone technique in predictive analytics. It provides a structured approach to understand relationships between variables. By creating mathematical representations, analysts can simulate real-world scenarios. A key characteristic of statistical modeling is its reliance on established mathematical principles. This makes it a trusted choice for many practitioners. However, while it offers high accuracy, it requires a solid understanding of statistics.
Machine Learning Algorithms
Machine learning algorithms significantly enhance predictive analytics capabilities. They continuously learn from data, improving predictions over time. This adaptability is a valuable feature that distinguishes machine learning. With their ability to analyze vast datasets, these algorithms can identify complex patterns that traditional methods may overlook. However, implementing machine learning requires technical expertise, which can present challenges for some organizations.
Data Mining
Data mining is another valuable technique in predictive analytics. It extracts patterns from large datasets through various methods, such as clustering and association rule mining. Data mining's key characteristic is its focus on discovery rather than prior hypotheses. This exploratory approach can reveal unexpected insights, making it instrumental in industries like retail and finance. However, the need for large amounts of data can be a limitation in its application.
Applications of Predictive Analytics
Marketing
Predictive analytics transforms marketing strategies by identifying potential customers and tailoring campaigns based on behavior. Its ability to segment target audiences and personalize offers is a significant advantage. Marketers can allocate resources more efficiently, ensuring maximum return on investment. However, reliance on algorithms can sometimes lead to overspending if not monitored closely.
Finance
In finance, predictive analytics helps assess risks, forecast market trends, and prevent fraud. This application is crucial for asset management and loan approval processes. The key characteristic is its ability to analyze real-time data. While it demands accurate data input for reliable predictions, the potential benefits include improved financial forecasting and risk management.
Healthcare
Healthcare is another field where predictive analytics proves beneficial. It helps predict patient outcomes, optimize treatment plans, and allocate resources effectively. A critical aspect of healthcare applications is the focus on patient data security and compliance. The advantages include better patient care and reduced operational costs. However, ethical considerations must always be a priority when dealing with sensitive health information.
Risk Management
Risk management heavily relies on predictive analytics to identify and mitigate potential threats. Organizations can analyze historical data to forecast future risks and develop proactive strategies. A key characteristic of these applications is their preventive nature, allowing businesses to save resources. The downside lies in data inaccuracies, which can lead to flawed risk assessments.
Interplay of Big Data and Predictive Analytics
The relationship between Big Data and predictive analytics is a convergence that reshapes industries and drives innovations. Understanding this interplay is crucial as organizations strive to leverage vast datasets to predict outcomes, optimize operations, and make informed decisions. The fusion of these two elements enables businesses to uncover hidden insights and identify opportunities that were previously unattainable.
Big Data acts as the raw fuel for predictive analytics, making it possible to develop models that anticipate future events. Instead of relying on smaller datasets with limited information, predictive analytics uses the volume, velocity, and variety of Big Data to produce more accurate and credible forecasts. This capability to analyze large chunks of data in real-time has turned into a game changer, especially for industries like finance, healthcare, and retail.
Moreover, integrating Big Data into predictive analytics enhances the granularity of insights. More granular insights can help organizations pivot strategies tailored to specific customer needs or market shifts. The overall outcome is a heightened ability to respond or adjust based on the analytical models derived from extensive data sets, thus increasing competitiveness.
This section details how Big Data empowers predictive analytics, along with the challenges that arise from their integration. Understanding these dynamics will enable IT professionals and decision-makers to harness the full potential of these technologies.
How Big Data Fuels Predictive Analytics
Big Data fuels predictive analytics through its diverse elements. First, large volumes of data provide a richer foundation for building models. Predictive models require substantial historical data to learn patterns and trends, making the vast volumes inherent in Big Data essential.
Additionally, diverse data types, such as structured, semi-structured, and unstructured data, help refine the models by introducing various dimensions of analysis. This variety introduces complexity that enhances model robustness. For example, Twitter data may help sentiment analysis in marketing, while transactional data from credit cards can focus on purchasing behavior analysis.
Having access to real-time data feeds allows predictive models to be continuously updated, improving accuracy over time. The ability to adapt to changes in data is a significant advantage of Big Data, making predictive analytics more relevant in dynamic environments.
Challenges in Integrating Big Data with Predictive Analytics
Despite the advantages, several challenges exist in integrating Big Data with predictive analytics. Addressing these challenges is essential for achieving optimal performance and reliable forecasts.
Data Quality Issues
Data quality is a chief concern that affects predictive analytics' reliability. Poor-quality data can lead to unreliable predictions, as the models rely on the integrity of the data being analyzed. For example, if the historical data used is inaccurate, the forecasts generated will almost certainly be flawed. Common issues include missing values, inconsistencies, and inaccuracies.
Ensuring data quality is a vital step toward leveraging Big Data efficiently. High-quality data also boosts the credibility of insights provided, making data quality a beneficial focus in this article.
Scalability Concerns
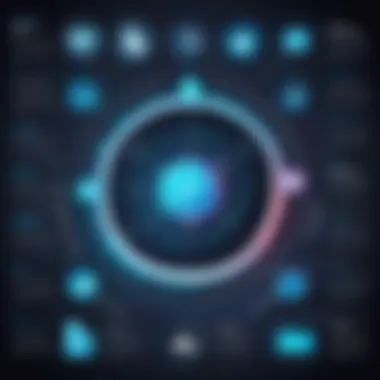
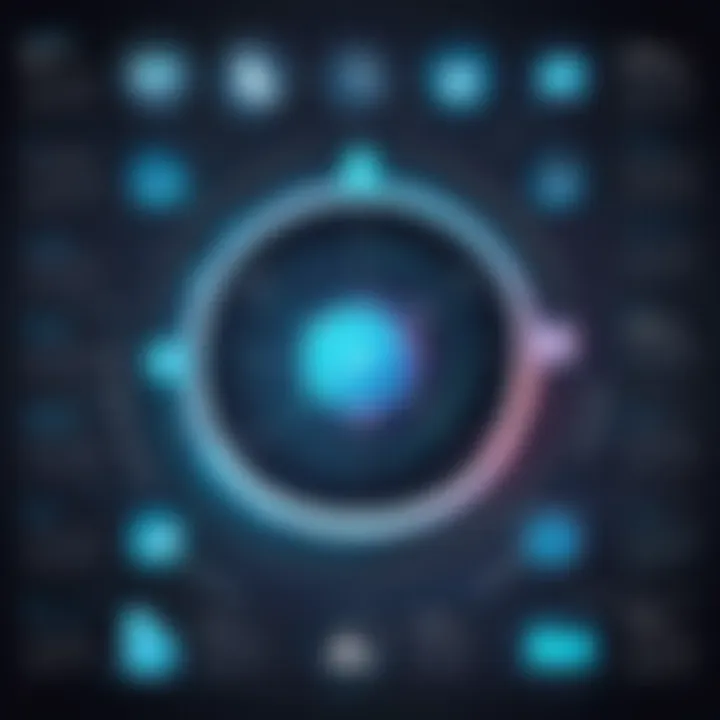
Scalability involves the ability of a system to handle increasing amounts of data without compromising performance. As the volume of data continues to grow exponentially, software and hardware infrastructures must adapt swiftly. Many organizations face limitations in their current data infrastructure.
Scalability must be factored in when developing predictive models and systems for handling Big Data. Proposed solutions must seamlessly manage or even increase their capacity, ensuring that performance remains constant even as data loads grow.
Interoperability
Interoperability concerns how well different systems and software can work together. In a landscape where organizations frequently employ multiple tools and platforms for data processing, the ability of these systems to integrate and communicate efficiently is vital. Silos can exist, preventing organizations from getting a complete picture of their data landscape. This lack of integration results in data inconsistencies and potential gaps in insights.
Thus, achieving interoperability is not only beneficial but necessary to fully utilize the potential of both Big Data and predictive analytics. Solutions that support data integration across platforms will enhance the overall effectiveness of analytical capabilities.
Data Management in Predictive Analytics
Effective data management is central to successful predictive analytics. As organizations collect vast amounts of data from various sources, the ability to manage that data becomes increasingly essential. Proper data management creates a robust foundation for analyzing data, driving informed insights, and making strategic decisions.
Data management in predictive analytics involves the processes of gathering, storing, organizing, and maintaining data. The quality of data directly impacts the accuracy of predictions made through analytics. Therefore, understanding the significance of data governance, data cleaning techniques, and the role of data warehousing is crucial for IT professionals.
Importance of Data Governance
Data governance is the framework that outlines how data is managed and used within an organization. It provides a set of policies and standards that ensure data accuracy, availability, and security. The primary objective of data governance is to maximize data's reliability and enforce compliance with regulations.
Having strong data governance measures is important for several reasons:
- Data Integrity: Ensures that data is accurate and trustworthy.
- Compliance: Helps organizations adhere to legal regulations.
- Decision-Making: Facilitates better decision-making processes by providing reliable data.
Implementing data governance in predictive analytics enables organizations to maintain high-quality data that enhances predictive accuracy, ultimately leading to more effective strategies.
Data Cleaning Techniques
Data cleaning is a vital part of data management. It involves identifying and correcting inaccuracies in the data. Several techniques help in cleansing data:
Normalization
Normalization refers to the process of organizing data to minimize redundancy and improve data integrity. The primary characteristic of normalization is its ability to streamline datasets. This makes it easier to perform analytics, such as predictive analytics.
Normalization is a popular choice because it enhances data consistency and helps reduce discrepancies. However, normalizing data can sometimes lead to complexity in querying the data, as it involves multiple tables.
Transformation
Transformation is the process of converting data into a more appropriate format or structure for analysis. This may involve converting data types, aggregating data, or combining multiple datasets. The key characteristic of transformation lies in its flexibility, as it allows adaptability for different analytical needs.
It is a beneficial approach because it enhances the capability of predictive models. However, transformation can introduce risk if not done carefully, potentially leading to data loss or misinterpretations.
Imputation
Imputation is a technique used to fill in missing values within a dataset. This is vital for maintaining the integrity of analyses, as missing data can skew results. The characteristic of imputation is based on statistical methods to estimate or predict missing data points.
Imputation is advantageous because it allows analysts to retain the dataset's size while mitigating its gaps. However, if done incorrectly, it can introduce bias into the data and affect predictive accuracy.
Role of Data Warehousing
Data warehousing serves as a centralized repository for data collected from different sources. It plays an essential role in predictive analytics by enabling seamless data access and management. The repository allows for effective querying, reporting, and analysis of large datasets.
A well-structured data warehouse supports complex analytical queries and improves data accessibility. It allows businesses to consolidate data and provide meaningful insights. However, implementing a data warehouse can be costly and requires careful planning to align with organizational needs.
Ethical Considerations in Big Data Usage
The use of big data brings substantial benefits, yet it prompts significant ethical questions. Understanding ethical considerations is crucial in ensuring responsible use of data across various sectors. This section discusses the importance of data privacy, ethical data use, and the implications of regulatory compliance. Addressing these areas helps to build trust and integrity in the field of big data and predictive analytics.
Data Privacy and Protection
Data privacy and protection are fundamental in the context of big data. Organizations must ensure the confidentiality of personal information and protect it from unauthorized access. This serves several purposes: it maintains user trust, adheres to legal requirements, and upholds corporate reputation. Data breaches can result in devastating financial and reputational consequences, making strong data protection measures not just necessary but essential.
Ethical Use of Data
Consent
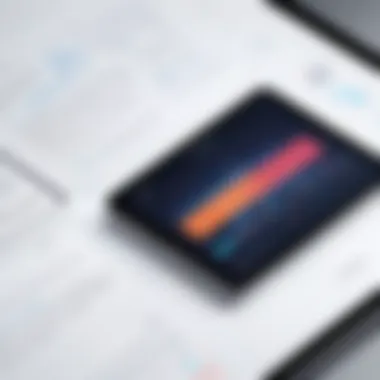
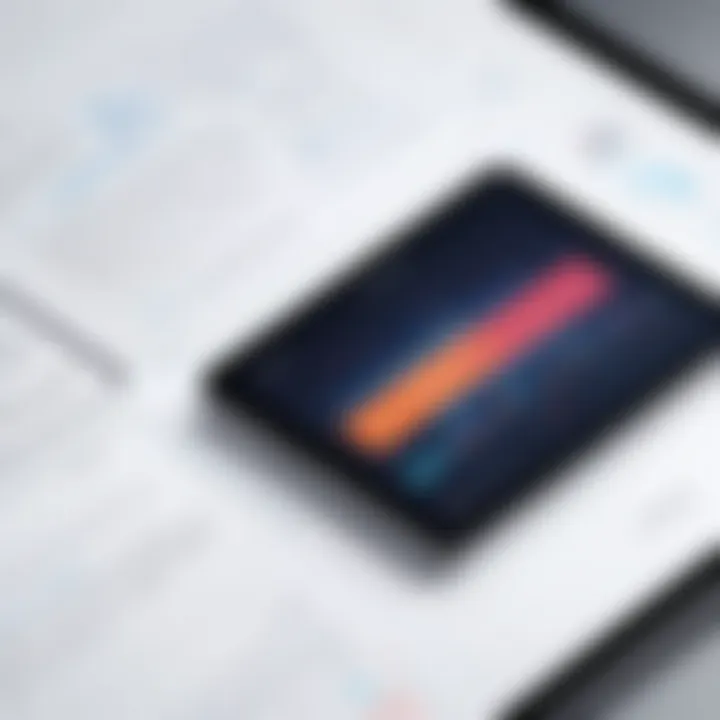
Consent is a touchstone in ethical data use. It requires organizations to obtain permission from individuals before collecting or processing their data. This practice respects privacy rights and fosters trust. If consent is obtained transparently, it contributes positively to the ethical landscape of data analytics. However, complexities arise in ensuring consent is truly informed, particularly in cases of extensive data collection.
Transparency
Transparency refers to the clarity with which organizations communicate their data usage intentions to consumers. Being transparent builds confidence. When individuals know how their data will be utilized, they are more likely to engage. However, achieving transparency can be difficult as organizations navigate nuanced data practices, which sometimes leads to misinterpretations.
Regulatory Compliance
Regulatory compliance plays an indispensable role in guiding ethical data practices. Organizations must navigate complex laws designed to protect consumer information. Failing to comply can lead to severe penalties.
GDPR
The General Data Protection Regulation (GDPR) establishes strict guidelines for data protection. Its focus on user consent and data rights ensures fair treatment. GDPR is widely recognized as a benchmark for privacy laws. Its rigorous requirements compel businesses to enhance their data handling processes, although compliance can be challenging and costly.
CCPA
The California Consumer Privacy Act (CCPA) enhances privacy rights for California residents. It emphasizes consumer empowerment by allowing individuals the right to know what data is collected and to whom it is sold. CCPA is increasingly relevant as more states adopt similar regulations. However, navigating compliance can be demanding, especially for smaller businesses.
Case Studies of Predictive Analytics in Action
Exploring case studies of predictive analytics is essential in understanding its practical applications and benefits across various sectors. These case studies serve not just as illustrations but also provide insights into the methodologies, technologies, and results achieved through predictive analytics. Analyzing real-world examples allows IT professionals and enthusiasts to see how theory translates into practice and the tangible outcomes organizations can achieve.
The examination of these case studies can help identify best practices, highlight common pitfalls, and demonstrate effective strategies for leveraging predictive analytics. These elements are crucial for decision-making, enhancing the ability to respond proactively rather than reactively. Using these insights can significantly improve operational efficiency, customer engagement, and overall performance.
"Real-world applications of predictive analytics can reveal what works and what doesn’t, offering invaluable lessons for those looking to implement these strategies."
Predictive Analytics in Healthcare
Predictive analytics has a transformative impact on the healthcare sector. Hospitals and healthcare providers are increasingly utilizing predictive models to forecast patient outcomes, optimize resource allocation, and enhance patient care. For instance, predictive analytics can identify high-risk patients, allowing preemptive action that can improve health outcomes and reduce costs.
One notable case is the use of predictive analytics in managing emergency room operations. By analyzing patient data and historical trends, hospitals can more accurately predict peak hours of patient influx. This enables them to adjust staffing levels and prepare resources accordingly, thereby minimizing wait times and improving patient satisfaction.
Moreover, predictive analytics assists in the development of personalized treatment plans. An analysis of vast datasets allows for the identification of patterns that can inform clinicians about the most effective treatments for individual patients, based on their unique circumstances. This tailored approach not only improves treatment effectiveness but also enhances overall patient engagement and adherence to treatment.
Predictive Analytics in Marketing
In marketing, predictive analytics is increasingly essential for crafting effective campaigns and strategies. Businesses harness customer data to discern patterns in purchasing behavior, allowing them to anticipate customer needs and preferences. A notable example involves large retailers who analyze purchase histories to recommend relevant products, resulting in higher conversion rates.
Predictive analytics also plays a critical role in customer segmentation. By employing clustering algorithms, organizations can divide their customer base into distinct groups based on behaviors and preferences. This targeted approach allows marketers to tailor their messages and offers more effectively, enhancing the likelihood of customer engagement.
Additionally, brands use predictive models to optimize their inventory management. By forecasting demand based on previous sales data, businesses can ensure that they maintain optimal stock levels, thus minimizing waste and maximizing profitability.
The benefits of using predictive analytics in marketing continue to expand as technology advances. Organizations that embrace these methods position themselves to respond dynamically to market shifts and consumer trends, creating a competitive edge.
Future Trends in Big Data and Predictive Analytics
The world of technology is always evolving. As big data and predictive analytics continue to grow, it is important to understand the future trends shaping these fields. These trends not only influence how businesses operate but also how they make critical decisions. By examining these elements, IT professionals can strategize effectively and stay ahead of the competition.
Emerging Technologies
Emerging technologies play a key role in the advancement of big data and predictive analytics. Innovations like blockchain, edge computing, and quantum computing are starting to reshape these fields significantly.
- Blockchain: This technology offers enhanced data security and transparency. For big data, it ensures that the integrity of data remains intact.
- Edge Computing: Handling data closer to the source minimizes latency and bandwidth use. This is essential for applications requiring real-time analytics.
- Quantum Computing: With its ability to process vast amounts of data quickly, quantum computing stands to revolutionize predictive analytics. It can analyze complex datasets much faster than classical computers.
These technologies combined will offer new capabilities that transform data processing, leading to more accurate and timely insights.
The Role of Artificial Intelligence
Artificial Intelligence (AI) is another driving force behind future trends in big data and predictive analytics. AI algorithms enhance the predictive capabilities by analyzing data at scale. Automation of data insights allows businesses to make more informed decisions.
Moreover, AI can improve data cleansing processes. For example, machine learning algorithms can identify inaccuracies in data sets autonomously. This means the models built on these datasets are likely to be more reliable. Companies can then focus on strategic initiatives rather than data management challenges. As AI continues to evolve, its implications for predictive analytics will grow exponentially.
Predictive Analytics in Real-Time Applications
Real-time analytics is becoming increasingly vital. Companies are shifting focus towards actionable insights delivered instantaneously. Areas like financial services, e-commerce, and healthcare benefit immensely from this trend.
In finance, real-time risk assessment not only improves transparency but also customer satisfaction. E-commerce platforms track consumer behavior as it happens, allowing for tailored marketing strategies that increase conversion rates.
In healthcare, real-time data analysis can provide immediate feedback on patient vital signs, which can be life-saving. Predictive models now play a central role in proactive treatment approaches instead of reactive measures.
The future of big data and predictive analytics remains bright, with emerging technologies, artificial intelligence, and real-time applications paving the way for smarter business decisions.